Revolutionizing Air Travel: New Turbulence Detection Method Unveiled
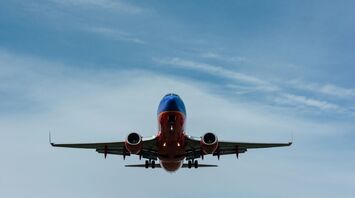
In a breakthrough development for aviation safety, a team of four aviation professionals from China has unveiled a new method for detecting turbulence anomalies. Published in the prestigious journal "Advances in Atmospheric Sciences," their research introduces a simplified approach leveraging quick access recorder (QAR) data to identify turbulence risks, promising to significantly enhance safety measures in civil aviation.
This new methodology, rooted in the use of genetic programming for symbolic classification, bypasses traditional turbulence assessment metrics, offering a more streamlined and efficient process. Turbulence, a major safety concern for airlines, manifests through sudden airflow changes that can lead to rapid, unpredictable aircraft movements. By utilizing data readily available from QARs installed on aircraft, this innovation allows for the early detection of turbulence anomalies without the complex computations previously required.
The significance of this development cannot be overstated. Turbulence poses not only a direct risk to passenger and crew safety but also affects flight operations and airline economics through potential injuries, medical emergencies, and the subsequent operational disruptions and financial losses. Furthermore, passenger confidence in air travel could be shaken by turbulence incidents, affecting customer satisfaction and loyalty.
According to Hongying Zhang, the corresponding author and a member of the Civil Aviation University of China, the availability of QAR data across modern aircraft makes their method universally applicable, marking a considerable advancement towards enhancing global aviation safety. Co-author Pak-Wai Chan, from the Hong Kong Observatory, highlights the potential of integrating symbolic classifiers into turbulence monitoring systems to refine detection processes and improve anomaly identification accuracy.
As the aviation industry grapples with the challenges posed by climate change and its own expansion, the necessity for effective turbulence monitoring and mitigation strategies has become increasingly pressing. The proposed method not only signifies a leap forward in addressing these needs but also sets the stage for future advancements in turbulence level classification and severity estimation through multi-classifiers and regression models.
This innovative approach holds the promise of transforming how the aviation sector manages turbulence risks, ultimately leading to safer skies for passengers and crew alike.